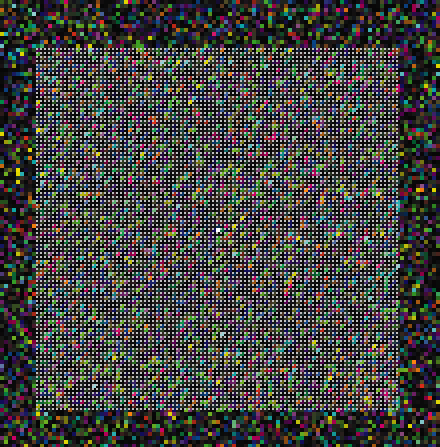
Pattern recognition on EEG customized equipment
By Constantin Costescu
Customized EEG gets data from human brain. These data and electrical impulse can be stored on vector or matrix.
Running pattern recognition on these vectors on different state as meditation, sleep, speaking, debating, etc and correlating this with patients markers will associate the found patterns with patient state.
The patient’s markers are what patient feels on a specific timestamp.
If the patterns found in different samples, are identical with the patient markers, we can assume that the patterns found will characterize the specific patient’s state.
Once we have these patterns found, we will consider that those patterns are the basic patterns.
Next step, and we now enter into the real problem, is to identify these patterns in other samples of data that we acquire with different methods of capturing uncommon brain signals.
Supposing we have the sample P00 that was obtained on Alpha9 (Alpha wave 9Hz), we will determine the dominant pattern P0; P1 from the samples P01 and so on.
(C0..Cp) is the total characteristics that we can deduct based on (P0..Pn).
(P00..Pkn) are samples acquired with different sensors, from different subjects, others than common one used to get (P0..Pn) patterns. For better results, the specific time stamp can be use.
We have the total of samples Pkn, with the dominant patterns Pn, n,k
The cutting EDGE is the new customized sensor that will be able to acquire signals (samples), that can be not just electrical but different forms of brain signaling.
What if the answer we are looking for is not in electric response but in other form of signaling, not electric, magnetic or electromagnetic?
Without patterns (P0..Pn) we will be blind searching for something. Fortunately, we have now P0 and that is giving us the chance to identify the basic patterns in the samples (P00..P0n), generalizing the patterns P0,P1..Pn will be obtained from (P00,..Pkn) data samples, n,k
For the next measurements, even we don’t know what is acquired, just running the pattern search algorithm, will suffice.
If a sequence Pm can be identified in (P01..P0n) interval, and this one is presenting the same characteristics as P0, then we can assume that the signal we acquired is the right one and we can go further with fine tuning.
In this way we will find the right signal that the brain is broadcasting, and we can step further to find or define another patterns Pj, that will be used for other procedures. j
Now we know where to find the matching patterns, in the source of new signal (samples) that brain broadcast, having the references (P1..Pn).
A new set of samples (P1n..Pnn) will be use to decode and to find the other information hidden in the brain broadcast data, and of course the new characteristics Cqp will be discovered. q,p
My goal is to find occurrence of P1..Pn patterns in the samples P1n.. Pnn, and more than that, to prove that the timestamp are identical reported to patient markers.
Once we can correlate the new patterns with patient markers we will have the data of patient markers. That means that we can identify the patient state looking to a data vector.
Gradually we will add new patient markers and find the specific data vectors for these markers.
Having the more patience markers and data we can identify a state or deseas in other patient based patient vector data flow.
When the datavector will be decoded we will have more hidden information about the patient.
Theorem: The sum of cdata obtained from basic patterns is less or equal than the sum of total cdata from determined patterns.
This theorem is the basement for medical investigations of the future.
The materials can be downloaded from Research Gate.
Jun 29, 2015, Constantin Costescu